That professional sports gain their unique charm from unusual situations where underdogs defeat the favourites. The professional teams serving smaller urban areas typically enter matchups against their big city rivals as the betting underdogs. The betting markets have shown a consistent error since the last decade through which small market teams regularly exceed predicted outcomes.
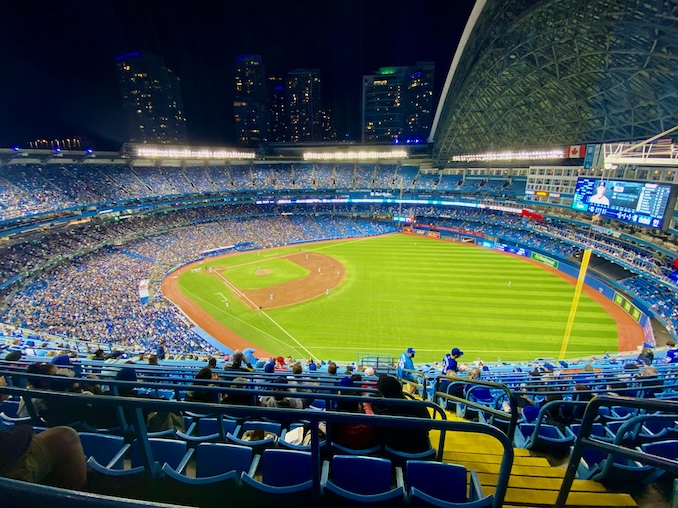
The Algorithm Bias Against Small Markets
Multiple components determine sports betting lines through advanced algorithms including statistical player data and injury updates alongside matchup history and meteorological elements. The mathematical prediction models demonstrate a hidden preference toward teams operating in larger market areas. The algorithm produces this bias unintentionally through its underestimation of factors that do not match the formulas it uses for evaluation.
Fanatics studying sports betting have started detecting this pattern most noticeable in MLB and NBA because of their large differences in market size. Professional bettors use tracking systems to find beneficial wagering possibilities through backing small market teams during their matches against established opponents. Through its focus on market size the algorithms create an inefficient trading condition that betting professionals exploit by correctly identifying discrepancies between fan interest and actual competitive dynamics.
Numerous studies from university-based sports economists prove the occurrence of this phenomenon. Researchers studied betting lines through major sports for five years and discovered that teams with less fan base beat greater market teams by 3.2% on average. The difference between actual winning outcomes and betting odds results in the annual loss of billions of wagering money.
The Human Element Behind the Numbers
The ongoing algorithmic prediction error mainly results from the approaches used by small market teams when constructing rosters and developing talent. Financial limitations force the teams to develop novel methods for talent identification as well as analytical tools. Through their strategic approach the Milwaukee Brewers developed an outstanding pitching program from resources with limited value in the marketplace. The Utah Jazz apply defensive strategies while they focus on metrics that the market overlooks. Such ground-breaking developments escape detection from national media organizations and end up shaping major outcome results. Computer programs cannot detect fundamental elements such as team unity which springs from a unified mentality of battling collectively against opponents. The strong sense of community bolsters home-field benefits beyond what regular statistical adjustments measure which strengthens the market disadvantage of small market teams.
Data Limitations and Media Influence
Data collection serves as a major contributing factor to how algorithms fail to identify certain game characteristics. Major market teams are analyzed in more detail than small market teams whose attributes frequently escape notice. The betting algorithms fail to represent small market strategies because these approaches receive minimal analysis. When media presents information they typically focus on team financial challenges instead of their competitive abilities. Algorithms that perform sentiment analysis detect media biases about small market teams which causes their betting values to decrease further.
Smart Money Follows the Pattern
Competent bettors find this unusual situation between large market teams and underdogs to be essential for developing profitable betting systems. They use recorded line movements and small market underdog results to take advantage of their systematic devaluation. Time has provided enough evidence to prove the inefficiency continues to exist. Industry analysts identify market segmentation as the cause of this trend because casual bettors prefer betting on big-market teams which forces bookmakers to adjust betting lines. The professional betting market remains unfavourable due to casual bettors who outnumber them. The algorithm fails to correctly predict small teams during entire playoff periods with even greater intensity. During their 2020-21 NBA postseason run the small-city Phoenix Suns proved betting predictions wrong multiple times.
Evolution of the Market
The market shows increasing efficiency because machine learning algorithms implement analysis from previous results. Sports analysis and sports journalism continue to have structural elements that cause the current level of market inefficiency. Modern bookmakers who aim to improve their services apply market-size adjustments to remedy this bias issue. Some bookmakers maintain secret strategies yet their approach changed since they faced many unsuccessful outcomes against professional bettors. The ongoing nature of this phenomenon challenges market efficiency together with behavioural bias. Complex AI-assisted models containing human-based decision parameters adopt human elements from their design structure and data choice processing. The small market bias clarifies how subjective cultural preconceptions impact statistical analysis systems.
Analysis of betting patterns uncover fundamental observations about sports and mathematical prediction systems because of steady low valuations toward teams in smaller markets. The cognitive biases integrate themselves effortlessly into supposedly objective systems to generate betting opportunities for smart gamers. Modern algorithms development demonstrates that mathematical interpretation of human competition remains imprecise even when using evolving mathematical systems.
For people who cheer for underdog teams there is beauty in the way their teams regularly overcome the margins between underdog and favourite. The financial gaps which favour opposing teams do not stop these underdog teams from challenging both the mathematical predictions alongside the established wagering odds. Sports continue to resist numerical prediction precision which keeps their competitions highly engaging for viewers.